Looking Into the Development of Artificial Intelligence with a Senior AI Researcher at Google DeepMind

Bandung, itb.ac.id—The Informatics Study Program of the School of Electrical Engineering and Informatics of Institut Teknologi Bandung (ITB) held a public lecture about Deep Learning and AlphaCode as the closure of IF2211 Algorithm Strategy Course on Wednesday (27/4/2022). This lecture was delivered by Adhiguna Surya Kuncoro.
Adhi is an Informatics alumnus of ITB from 2009. He is a senior artificial intelligence (AI) researcher at Google DeepMind. The public lecture was moderated by Dr. Ir. Rinaldi Munir, M.T., and was conducted through Zoom for about 90 minutes.
Adhi began his lecture by explaining the scientific field of artificial intelligence. AI is a field of study that aims to create intelligent machines that have the intelligence of a human. One example is AlphaGo Zero, a product of Deepmind. AlphaGo Zero is an AI that is capable enough to beat the world champions of Go after a measly training time of 4 hours. This is really astonishing considering that Go itself is not a simple game. One problem is the sheer number of possible steps, which is about 10 to the 17th power, more than the number of atoms in the universe.
Another example would be the use of AI in solving algorithmic problems, or Alphacode to be exact. According to Adhi, a research on AI like this is important since it can test the machine’s understanding of the human language. Doing competitive programming is just basically the same as translating the human language (the question) to the programming language (the answer).
The performance of the AI model is tested on a competitive-programming platform that many people use, Codeforces. The results of the contestants from the last six months show that AlphaCode is at the top 28% of the ranking. This means that the AI’s performance surpasses the average of Codeforces users (>50%).
AlphaCode’s own architecture model is Transformers, the same architecture used in Google Translate. Technically, in the first phase, pre-training is conducted on the model. The purpose is to familiarize the model with the semantic context of a code—the model does not learn from scratch—when it continues to the next phase. In the second phase, fine-tuning is performed so that the model can learn how to solve algorithmic problems. Upon completion, the AI program will execute a searching process (samples). For example, it filters the best 10 possible answers from a total of 10000 solutions.
*To see the live visualization and demonstration of AlphaCode, please visit this link: https://alphacode.deepmind.com/.
Even though the development of AI has been comparatively advanced, lots of good data are still needed to obtain a desirable AI performance. In this context, “good data” means appropriate and unbiased data. If the quality of the data is poor or inappropriate, the prediction results of the AI model may be inaccurate.
To illustrate the problem, Adhi used employee salary dataset as an example. This data cannot be used for AI models related to MSME income. This is because MSME income may be unstable, in contrast to employee income that tends to be stable. On the other hand, MSME income data may not be available in large numbers, hence it cannot be used to create a good AI.
For closing, Adhi said that there are many other technological developments that can still be explored. AI technology should be able to help many people around the world, not just a few individuals, and it can be utilized for tackling various issues such as vaccines, tropical climates, irrigation systems, and wildfires.
Reporter: Maria Khelli (Informatics Engineering 2020)
Translator: Ariq Ramadhan Teruna (Faculty of Industrial Technology, 2021)
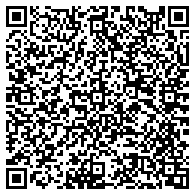
scan for download